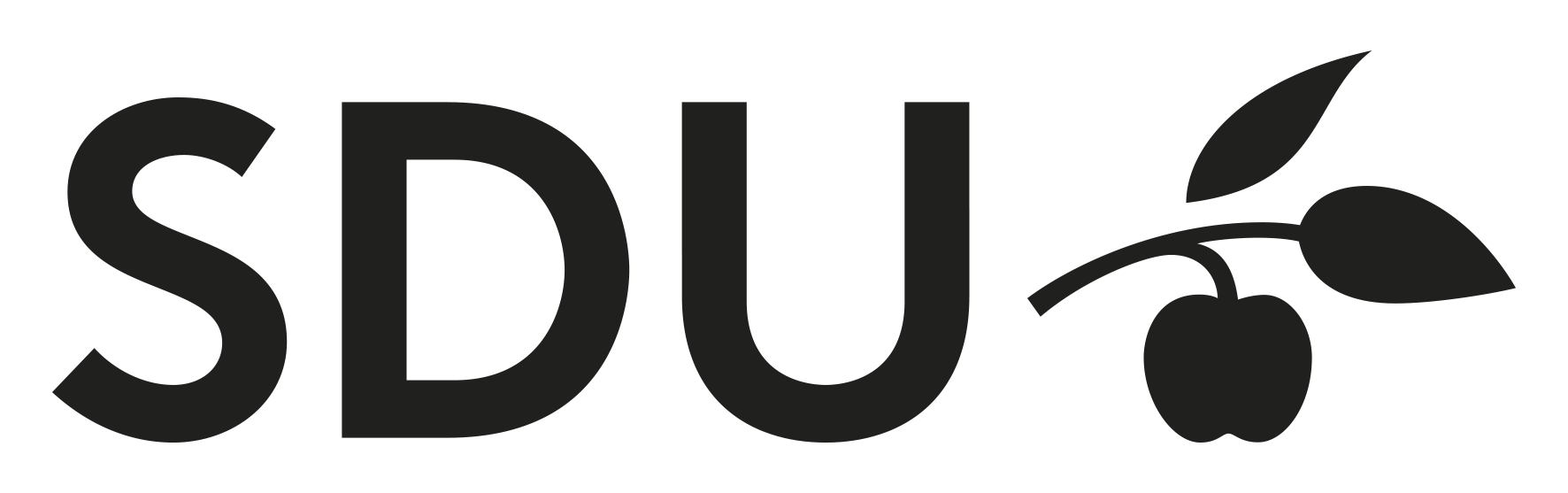
MM852: Computational option pricing, part 1
Study Board of Science
Teaching language: Danish or English depending on the teacher, but English if international students are enrolled
EKA: N310049102
Assessment: Second examiner: None
Grading: Pass/Fail
Offered in: Odense
Offered in: Spring
Level: Master
STADS ID (UVA): N310049101
ECTS value: 5
Date of Approval: 31-10-2023
Duration: 1 semester
Version: Approved - active
Entry requirements
This course cannot be taken in addition to MM542 or MM833, or if MM566 has been followed in spring 2024.
Academic preconditions
Academic preconditions. Students taking the course are expected to:
a) Have knowledge of at least one of the following subjects:
- Numerical solution of ordinary differential equations
- Theory of stochastic integration and stochastic differential equations
- Theory of option pricing
b) Be able to use Matlab or R.
Course introduction
The aim of the course is to enable the student to
- Model problems from financial mathematics, especially option pricing, by stochastic and partial differential equations,
- Solve the resulting problem classes efficiently by computational methods based on a sound mathematical analysis,
- Evaluate and select among the methodologies, tools and general skills of the subject area,
- Initiate independently and carry out interdisciplinary collaboration and assume professional responsibility,
- Independently take responsibility for his own professional development and specialisation.
The course builds on the knowledge acquired in the courses MM534 “Differential equations, Computing and Modelling”/ MM547 “Ordinary differential equations: Theory, Modelling and Simulation” or MM802 "Stochastic differential equations 1", and gives an academic basis for a Master project in several core areas of Natural Sciences.
In relation to the competence profile of the degree it is the explicit focus of the course to
- Give the competence to handle complex and development-oriented situations in study and work contexts.
- Give skills to:
- analyse practical and theoretical problems with the help of numerical simulation based on a suitable mathematical model
- describe and evaluate sources of error for the modelling and calculation of a given problem
- justify relevant models for analysis and solution and choose between them
- Give knowledge and understanding of:
- Mathematical modelling and numerical analysis in finance
- reflection on theories, methods and practices in the field of applied mathematics
Expected learning outcome
The learning objective of the course is that the student demonstrates the ability to:
- Deal with stochastic differential equation models in finance
- Analyse and simulate stochastic differential equations using appropriate, advanced methods and modern software
- Design and perform reliable simulations of stochastic differential equation model
Content
The following main topics are contained in the course:
- Numerical solution of stochastic differential equations
- Monte Carlo simulation of stochastic differential equations, with focus on variance reduction methods, especially Multilevel Monte Carlo
- Application to European, Asian, lookback, barrier and digital options
Literature
Examination regulations
Exam element a)
Timing
Spring
Tests
Project
EKA
N310049102
Assessment
Second examiner: None
Grading
Pass/Fail
Identification
Full name and SDU username
Language
Normally, the same as teaching language
Examination aids
To be announced during the course
ECTS value
5
Indicative number of lessons
Teaching Method
To enable the students to reach the learning goals, the teaching is organized such that there will be 4 confrontation hours per week during 7 weeks. These teaching activities will correspond to an expected distribution of the work load for an average students as follows:
- Aktivity - Number of hours:
- Intro phase - Number of hours: 14
- Training phase - Number of hours: 14
- Study phase: Number of hours: 70
- Total: Number of hours: 125
Teaching is centred on interaction and dialogue. In the intro phase, concepts, theories and models are introduced and put into perspective. In the training phase, students train their skills through exercises and dig deeper into the subject matter. In the study phase, students gain academic, personal and social experiences that consolidate and further develop their scientific proficiency. Focus is on immersion, understanding, and development of collaborative skills.
Study phase acitivities:
- Reading suggested literature
- Preparation of exercises in study groups
- Contributing to online learning activities related to the course.
Teacher responsible
Timetable
Administrative Unit
Team at Educational Law & Registration
Offered in
Recommended course of study
Transition rules
Transitional arrangements describe how a course replaces another course when changes are made to the course of study.
If a transitional arrangement has been made for a course, it will be stated in the list.
See transitional arrangements for all courses at the Faculty of Science.