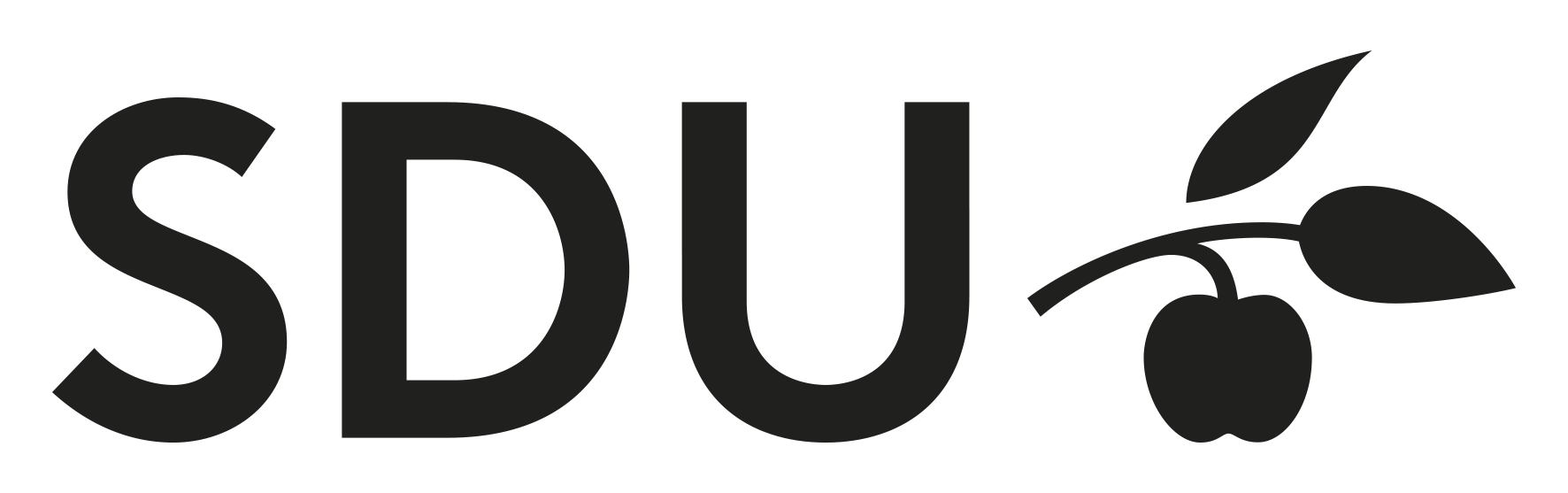
DS824: Fra Data til Evidens
Entry requirements
This course cannot be taken by students enrolled in the Bachelor - or Master program in Computer Science.
Academic preconditions
Students taking the course are expected to:
- Have knowledge of machine learning and artificial intelligence, e.g. from the mandatory courses in the master program in Data Science
- Have knowledge of fundamental statistics, e.g. from the mandatory courses in the master program in Data Science.
The student should bring a laptop to all lectures and the software STATA should be installed (download via your study page at mitsdu.dk ). Students who wish to use other software programs should make sure to have this installed and should be able to learn to program basic causal models in the given software on their own.
Course introduction
Objectives
The health care sector is based on evidence. Hence, new technology and new treatments will only be implemented if there is evidence of health benefits. This means that causal inference if of great importance. The objective of the course is to enable the student to understand the role of evidence in health care and the methods used to study causality. This is important to make the student capable of assessing when new technology, including artificial intelligence, can be expected to be implemented in health care.
- understaning and disentangling the three topics in Health Data Science (data description, prediction, causal inference)
- discussing pro- and cons of various methods to study causality and know the assumptions behind each method
- using the evidence term to assess possibilities and barriers for the use of artificial intelligence in health care
- understand the basic programming of causal models ind state (interrupted times series, regression discontinuity, difference in difference, instrumental variables)
- Knowledge about the needs and opportunities of the specialization when working with and processing data
- Competences in selecting, applying, and combining the right programming, statistics, and machine learning tools and methods to work with data relevant for the specialization
- Skills in managing complex work and development situations in the areas of data processing and analysis as well as starting up and executing analyses
Expected learning outcome
- explain the topics in Health Data Science
- understand the difference between association and causality
- explain for the main assumptions behind models of causality using observational data
- understand the demand for evidence in health care
Content
Health Data Science consist of three topic; descriptive statistics, prediction and causal inference. The course will introduce the concept of Health Data Science by placing the achieved competences in descriptive statistics and prediction (AI and machine learning) into the context of Health Data Science. With respect to programming skills the focus will be on statistical models for causal inference.
The following main topics are contained in the course:
- Part 1: Introduction to Health Data Science
- Part 2: Methods for causal inference
- Part 3: Use og AI in health care
Literature
Examination regulations
Exam element a)
Timing
Tests
Oral examination
EKA
Assessment
Grading
Identification
Language
Examination aids
To be announced during the course.
ECTS value
Additional information
Indicative number of lessons
Teaching Method
- Intro phase: 16 hours (lectures)
- Skills training phase: 8 hours (state training)
- self study of certain topics
- independent work with topics in the intro and study phase
- Preparation of synopsis