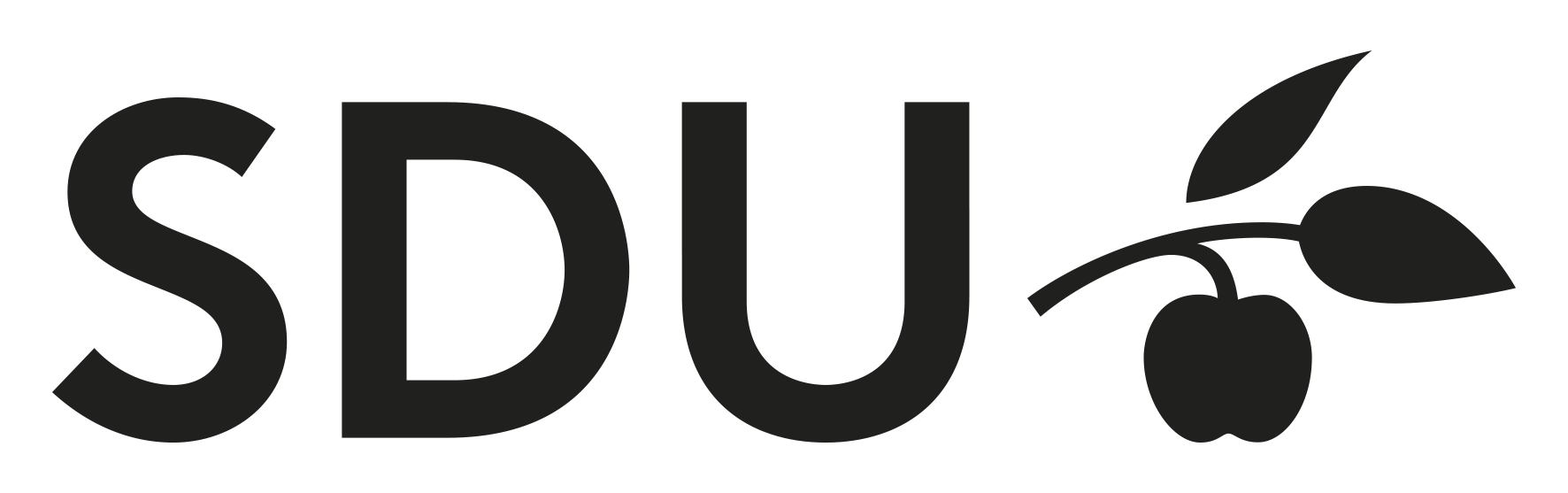
MM836: Convex analysis
Study Board of Science
Teaching language: Danish or English depending on the teacher, but English if international students are enrolled
EKA: N310051112, N310051102
Assessment: Second examiner: None, Second examiner: External
Grading: Pass/Fail, 7-point grading scale
Offered in: Odense
Offered in: Spring
Level: Master
STADS ID (UVA): N310051101
ECTS value: 5
Date of Approval: 01-11-2022
Duration: 1 semester
Version: Archive
Comment
Entry requirements
Academic preconditions
Students taking the course are expected to:
- Be familiar
with: systems of linear equations, matrices, determinants, vector
spaces, scalar product and orthogonality, linear transformations,
eigenvectors and eigenvalues, polynomials, the concept of a function and
its derivatives, real numbers, vector calculus.
Course introduction
The course will introduce analytic techniques and geometrical concepts
in order to solve linear and non-linear optimization problems, mostly
in economy.
in order to solve linear and non-linear optimization problems, mostly
in economy.
The course builds on the knowledge acquired in
the courses MM505 Linear Algebra, or MM540, or MM538, and MM533
Mathematical and Numerical Analysis.
the courses MM505 Linear Algebra, or MM540, or MM538, and MM533
Mathematical and Numerical Analysis.
The course is of high
multidisciplinary value and gives an academic basis for a Bachelor
Project in several core areas of Natural Sciences and Economy.
multidisciplinary value and gives an academic basis for a Bachelor
Project in several core areas of Natural Sciences and Economy.
In relation to the competence profile of the degree it is the explicit focus of the course to:
- Give the competence to :handle complex and development-oriented situations in study and work contexts.
Give skills to:
- apply the thinking and terminology from the subject's basic disciplines.
- analyze and evaluate the theoretical and practical problems for the application of a suitable mathematical model.
Give knowledge and understanding of:
- basic knowledge generation, theory and methods in mathematics
- how to conduct analyses using mathematical methods and critically evaluate scientific theories and models.
Expected learning outcome
The learning objectives of the course is that the student demonstrates the ability to:
- Correctly answer written assignments and prove results within the syllabus of the course.
- Reproduce and illustrate definitions and results within the syllabus of the course.
- Formulate answers to written assignments in a mathematically correct language.
- Give arguments for the steps in the solution of the exercises.
- Compare key results within the syllabus of the course.
- Understand and identify the practical problems that can be solved with the methods in the course syllabus.
- Use the presented methods to solve practical optimization problems.
Content
The following main topics are contained in the course:
Convex
sets and their topology, convex functions, conjugation,
subdifferentiability, minimization, Kuhn-Tucker theory, Numerical
optimization methods.
sets and their topology, convex functions, conjugation,
subdifferentiability, minimization, Kuhn-Tucker theory, Numerical
optimization methods.
Literature
Examination regulations
Exam element a)
Timing
Spring
Tests
Mandatory assignments
EKA
N310051112
Assessment
Second examiner: None
Grading
Pass/Fail
Identification
Full name and SDU username
Language
Normally, the same as teaching language
Examination aids
To be announced during the course
ECTS value
1
Exam element b)
Timing
June
Tests
Oral exam
EKA
N310051102
Assessment
Second examiner: External
Grading
7-point grading scale
Identification
Student Identification Card
Language
Normally, the same as teaching language
Duration
30 minutes
Examination aids
To be announced during the course
ECTS value
4
Indicative number of lessons
Teaching Method
Teaching activities are reflected in an estimated allocation of the workload of an average student as follows:
- Intro phase (lectures) - 28 hours
- Training phase: 14 hours
The intro phase will introduce general concepts and theory and exercise sessions will be devoted to learn material in depth. Interactive teaching will be used.
Educational activities
- preparation of exercises in study groups
- preparation of projects
Teacher responsible
Timetable
Administrative Unit
Team at Educational Law & Registration
Offered in
Recommended course of study
Transition rules
Transitional arrangements describe how a course replaces another course when changes are made to the course of study.
If a transitional arrangement has been made for a course, it will be stated in the list.
See transitional arrangements for all courses at the Faculty of Science.