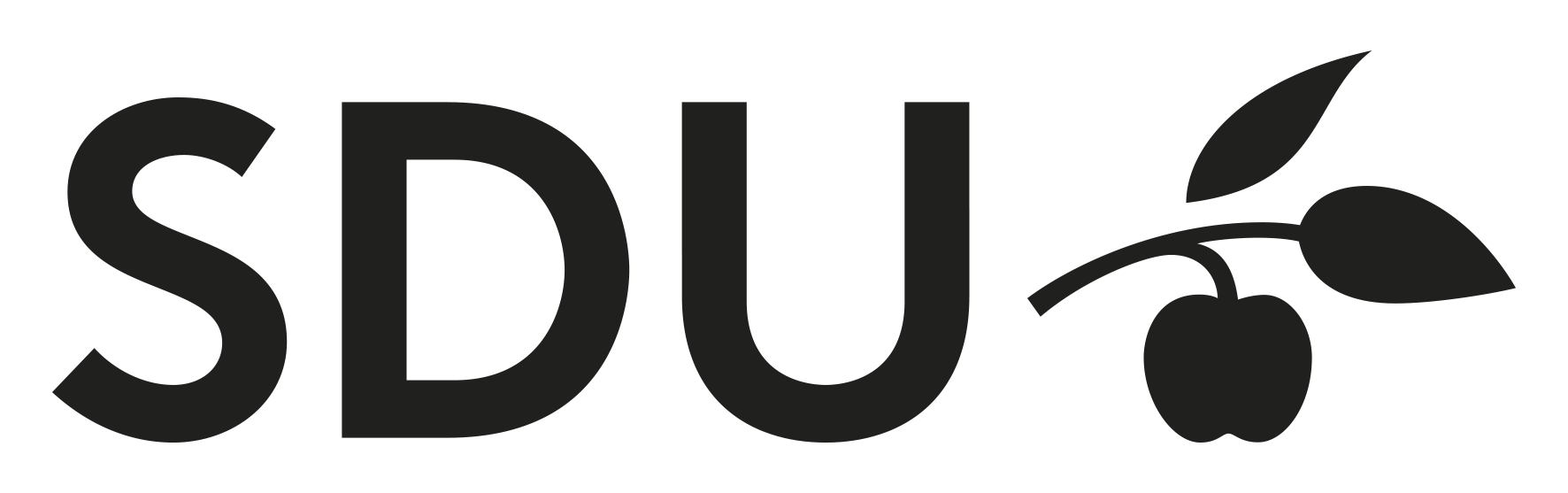
DS839: Causal Analysis in Business Data Science
Study Board of Science
Teaching language: Danish or English depending on the teacher
EKA: N340132102
Assessment: Second examiner: Internal
Grading: 7-point grading scale
Offered in: Odense
Offered in: Spring
Level: Master
STADS ID (UVA): N340132101
ECTS value: 5
Date of Approval: 30-10-2023
Duration: 1 semester
Version: Approved - active
Entry requirements
Academic preconditions
Familiarity with statistical analysis (DS803 and DS810). Knowledge of the software R is recommended but not required.
Course introduction
The main purpose of the course is to introduce students to causal analysis with a particular focus on its applications to data driven decision making.
The course will provide the student with knowledge about the central methods to identify and estimate causal effect and their intersection with big data analytics.
The students will have the skills to apply these methods to solve relevant empirical problems.
The course will give the students the competence to inform policy makers and businesses in relation to a wide range of problems that require to establish casual reletionships.
In relation to the competence profile of the degree it is the explicit focus of the course to:
•Give knowledge about and practical experience with the application of basic and advanced causal and machine learning methods and models
•Give competence to apply learned methods to concrete problems
•Give competence to illuminate hypotheses on a qualified theoretical background
•Give competence to promote collaboration and communication between data science and domain experts
The course builds upon knowledge from the course DS803 Statistics for Data Science as well as DS810 Data Driven Decision Making. The course provides a basis for possible thesis topics within causuality and machine learning.
Expected learning outcome
To fulfil the purposes of the course the student must be able to demonstrate knowledge about the course’s focus areas enabling the student to:
- Describe, explain and apply the different methods/models covered.
- Describe and explain how the different models are estimated.
- Describe and explain the difference between correlation and causation.
Content
1. Introduction to causal inference: potential outcomes and DAGs
2. Intersection between causal inference and machine learning
3. Advanced causal inference methods
Literature
Examination regulations
Exam element a)
Timing
June
Tests
Take home assignment
EKA
N340132102
Assessment
Second examiner: Internal
Grading
7-point grading scale
Identification
Student Identification Card - Exam number
Language
Normally, the same as teaching language
Duration
72 hour take home exam
Examination aids
All aids allowed
ECTS value
5
Additional information
The assignement is handed in, in Digital Exam.
Indicative number of lessons
Teaching Method
At the faculty of science, teaching is organized after the three-phase model i.e. intro, training and study phase. These teaching activities are reflected in an estimated allocation of the workload of an average student as follows:
Intro and training phases: 21 hours (divided approximately evenly into lectures and exercises)
Activities during the study phase:
Reading of course material
Reflection on methods and theoretical concepts
Solving selected exercises in R
Preparing a group presentation
Preparation for lectures
Preparation for exam
Teacher responsible
Name | Department | |
---|---|---|
Christian Møller Dahl | cmd@sam.sdu.dk | Econometrics and Data Science |
Giovanni Mellace | giome@sam.sdu.dk | Econometrics and Data Science |
Timetable
Administrative Unit
Team at Educational Law & Registration
Offered in
Recommended course of study
Transition rules
Transitional arrangements describe how a course replaces another course when changes are made to the course of study.
If a transitional arrangement has been made for a course, it will be stated in the list.
See transitional arrangements for all courses at the Faculty of Science.